BayVFP-Project KIProQua
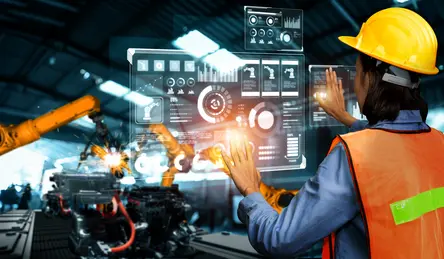
The project is a collaboration of the BMW Group and the University of Bamberg. We research novel AI-driven approaches for root cause analysis in the manufacturing process of electric vehicles. Our aim is to combine machine learning methods and symbolic AI to incorporate interactively expert knowledge into data-driven root cause analysis methods.
A human-in-the-loop approach to machine learning shall enable the identification of complex cause-effect relationships for product quality that go beyond current approaches of purely statistical anomaly detection in individual process sections. Through explicit use of expert knowledge as well as interactive corrections, adaptive quality models are developed that not only use local information but also map complex relational dependencies and thus contribute to the global optimization of process control. Through approaches of explainable AI (XAI), transparency and comprehensibility of the learned models is guaranteed. Thus, a contribution to acceptance and justified trust in the AI system is created. As an exemplary use case, the manufacturing process of the electric vehicles is considered. The goal is to develop an interactive dashboard based on innovative methods of explanatory and interactive learning. This enables the recognition of complex interdependencies in quality assessment and consequently increases production efficiency and minimizes rejects.
Publications and Talks
Publications
- Schramm, S., Wehner, C., Schmid, U. (2023). Comprehensible Artificial Intelligence on Knowledge Graphs: A Survey. In: Journal of Web Semantics, https://doi.org/10.1016/j.websem.2023.100806
- Wehner, C., Kertel, M., Wewerka, J.(2023). Interactive and Intelligent Root Cause Analysis in Manufacturing with Causal Bayesian Networks and Knowledge Graphs.In: IEEE 97th Vehicular Technology Conference (VTC2023-Spring), Florence, Italy, 2023, pp. 1-7, https://doi.org/10.1109/VTC2023-Spring57618.2023.10199563
- Eirich, J., Jäckle, D., Sedlmair, M., Wehner, C., Schmid, U., Bernard, J., Schreck, T. (2023). ManuKnowVis: How to Support Different User Groups in Contextualizing and Leveraging Knowledge Repositories. In: IEEE Transactions on Visualization and Computer Graphics, https://doi.org/10.1109/TVCG.2023.3279857
- Poßner, L., Bahr, L., Röhl, L., Wehner, C., Gröger, S. (2025). Anwendung von Causal-Discovery-Algorithmen zur Root-Cause-Analyse in der Fahrzeugmontage. In: Jochem, R., Meyer, M. (eds) Rethinking Quality - Wandel des Qualitätsmanagements durch Digitalisierung und Künstliche Intelligenz, GQWT24 2024, Springer Vieweg, Wiesbaden, https://doi.org/10.1007/978-3-658-47213-9_3
- Bahr, L., Wehner, C., Wewerka, J., Bittencourt, J., Schmid, U., Ruediger, D. (2025). Knowledge graph enhanced retrieval-augmented generation for failure mode and effects analysis. In: Journal of Industrial Information Integration, https://doi.org/10.1016/j.jii.2025.100807
Talks
- Wehner, C. (2022). Interactive and Explainable Link Prediction in Knowledge Graphs. Pattern Recognition and Artificial Intelligence. ICPRAI 2022. Doctoral Consortium.