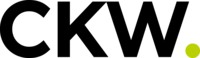


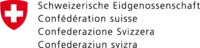
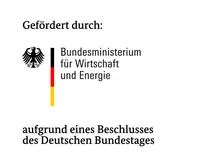
ERA-Net SG+ Project SmartLoad – Smart Meter Data Analytics for Enhanced Energy Efficiency in the Residential Sector
The arising smart metering infrastructure generates large amounts of data about energy consumption in the residential sector. This data contains extensive (hidden) evidence of household characteristics such as saving potential, suitability for self-supply and storage.
Based on ordinary 15-min smart meter data and further external information (e.g. geographical, socio-economical, weather, statistics), we develop and test methods to automatically derive individual household characteristics in this project. We focus on household characteristics in the field of energy efficiency (e.g. interest on renewables like eco-tarrifs for electricity) and the suitability of households for self-supply and storage. Furthermore, we will identfiy atypical consumption patterns, estimate base load, and aim to discover individual saving and load shifting potential.
Together with our implementation partner BEN Energy, we develop algorithms with smart meter data from the utility CKW, our industry partner, and test them in field. The realiziation of the project will follow Swiss and European data protection regulations.
We expect validated and highly scalable methods as a project result that discover energy saving potential in the residential area that helps utilities to improve their sales strategy for green and sustainable products in line with national energy strategies.
Research staff at the University of Bamberg: Andreas Weigert, Dr. Konstantin Hopf
Duration: 01.06.2017 — 31.03.2020
Total Budget: 802'378 € (industry and public funding)
Project Partners:
- Centralschweizerische Kraftwerke (CKW) AG, CH
- BEN Energy AG, CH
- Information Systems and Energy Efficient Systems, University of Bamberg, DE
Funding:
Selected Research Projects
Energy Data Analytics: Increasing Service Quality and Energy Efficiency in the Residential Sector
Utilities have a large customer base, yet their knowledge about individual households is small. This adversely affects both the development of innovative, household specific services and the utilities’ monetary KPIs. Our software solutions help utility companies to engage their customers in energy saving campaigns and support them in selling respective services.
In this project, we develop further and test in field experiments machine learning algorithms that infer household characteristics (apartment size, number of inhabitants and appliances, etc.) and predict the willingness to participate in efficiency or load shifting campaigns from load profiles, location information, and other existing customer data. Our tools provide customer insights at low cost and at scale, thereby solving an eminent business problem, improving the effectiveness of energy conservation campaigns, and ultimately increasing the customer value and adoption of related services.
Funded by the EU Eurostars Programme
Research staff at the University of Bamberg: Konstantin Hopf
Project duration: 01.11.2015 - 30.10.2018
Project Partners:
Total Budget: 818'840 € (industry and public funding)
Smart Meter Data Analytics for the Mass Market Energy Services
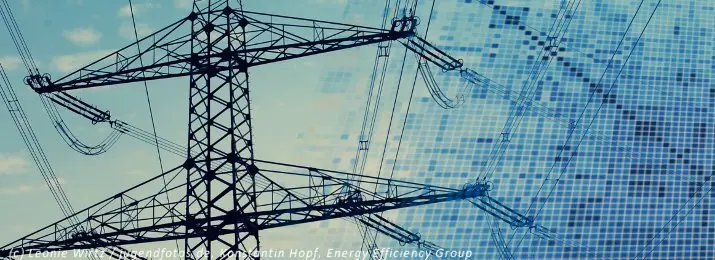
Smart electricity meters allow capturing consumption load profiles of residential buildings. The goal of the project is to develop methods of machine learning that will automatically infer energy efficiency relevant household characteristics (e.g., type of heating, living area, number of inhabitants per dwelling, etc.) from the power load traces. The gained information can be used by utilities as a starting point for developing energy consulting services, and for optimisation of sales channels.
The project will result to a new methodology and a software that will be employed by the customers of our partner BEN Energy already in 2016.

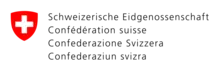

Funded by Commission for Technology and Innovation, Switzerland
Research staff at the University of Bamberg: Konstantin Hopf, Ilya Kozlovskiy, Dr. Mariya Sodenkamp
Project duration: 01.05.2014 - 30.04.2016
Project Partners:
- Chair of Information Management, Departement of Management, Technology, and Economics, ETH Zürich
- BEN Energy AG
Budget: CHF 442'560
Smart Meter Data Analytics for Automated Energy Consulting
Smart electricity meters allow for capturing consumption data of individual households at a high resolution in time (typically with 15-minute intervals). The key objective of this project is to develop further and evaluate feature extraction and machine learning techniques for automatic identification of household properties based on electricity load profiles. The gained information shall render highly targeted and scalable energy efficiency services possible.
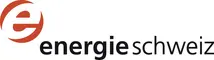
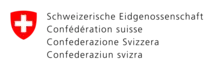
Funded by Swiss Federal Office of Energy and Arbon Energie AG, Switzerland
Research staff at the University of Bamberg: Konstantin Hopf, Ilya Kozlovskiy, Dr. Mariya Sodenkamp
Project duration: 01.04.2014 - 31.01.2016
Project Partners:
- Arbon Energie AG, Schweiz
- Chair of Information Management, Department of Management, Technology, and Economics, ETH Zurich
- Department of Computer Science, Institute for Pervasive Computing, ETH Zurich
Budget: CHF 353'450
DAIAD
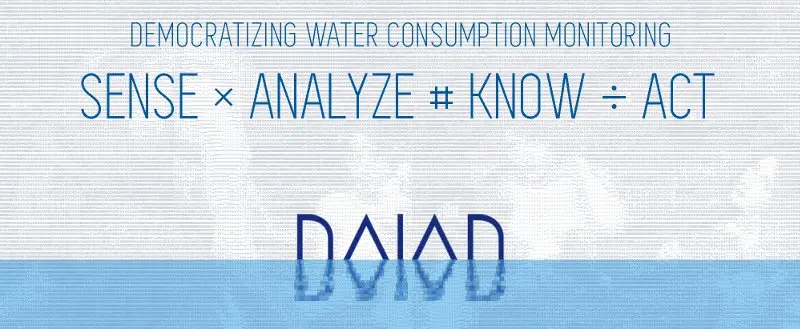
The EU Project DAIAD deals with the structure and the testing of an IT infrastructure in order to capture and analyze the consumption of (warm) water in private households. In association with Amphiro AG (CH), WaterWise (UK), the Athena Research (GR), the Frauenhofer Institute for “Systems and Innovation Research” and the City of Athens, the research group from Bamberg tests concepts for motivating sustainable consumer behavior.
Funded by EUs Seventh Framework Programme for Research
Project coordinator: Prof. Dr. Thorsten Staake ; Dipl.-Kffr. Anna Kupfer
Project duration: 01.03.2014 - 31.08.2017
Project Partner:
Budget: EUR 3'202'288
Data Analytics for Customer Segmentation and Engagement
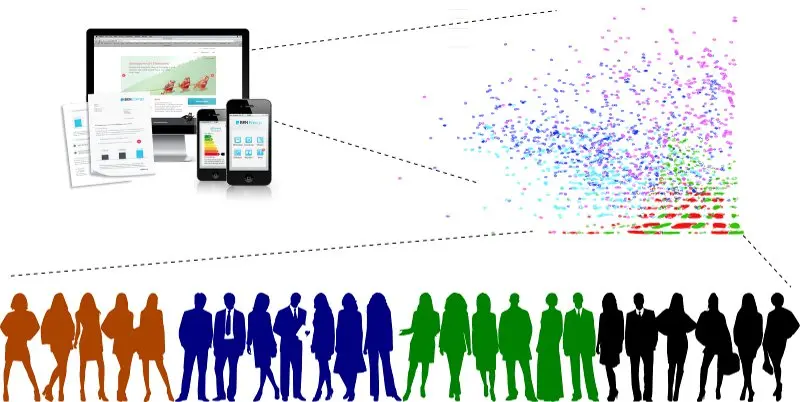
Customer satisfaction and confidence are the corner stones of long-term, successful and sustainable relationships between utility companies and energy consumers. The goal of the project is to develop methods for analysis of consumption and behavioral data that enable segmentation of end energy consumers in the residential sector. The derived information serves utility companies as a basis for targeted efficiency interventions and confidence building dialog with customers by intelligent linking of customer data.

Funded by RWE AG
Research staff at the University of Bamberg: Ilya Kozlovskiy, Dr. Mariya Sodenkamp
Project duration: 01.10.2013 - 31.03.2015
Projektpartner: BEN Energy AG
Sense4EN
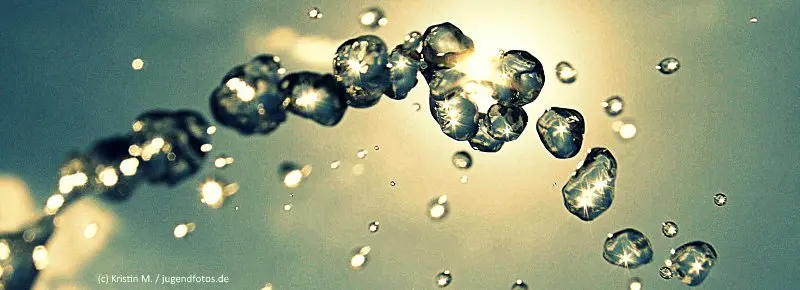
The project Sense4EN is funded by the European Union and part of the European ClimateKIC initiative. The Energy Efficient Systems Group elaborates concepts for reducing heat consumption (especially warm water) by means of Smart Metering systems in association with ETH Zurich, TU Berlin and Vattenfall.